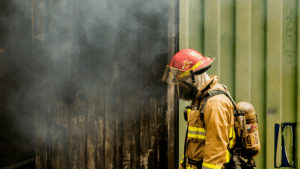
- July 1, 2025 3:46 pm
- California
Over the past three years, participants in consumer finance, including originators, investors, and servicers, have enjoyed a prosperous period. With numerous forms of government stimulus, such as low interest rates, rental assistance, mortgage forbearance, student loan forbearance, tax credits, and individual stimulus checks, US consumers found themselves with more liquidity than usual, resulting in an incredible multi-year stretch of outstanding performance for consumer loan portfolios.
However, by early 2022, the Federal Reserve had to take action as inflation was increasing exponentially, and interest rates were raised to combat this.
Around this time, consumer loan delinquency rates began to increase for the first time in years, causing servicers to revisit their loss mitigation playbooks, and portfolio owners to become nervous.
At Goal Solutions, we have faced similar economic shifts before, as we built our master servicing business in the wake of the Great Financial Crisis of 2008-2009. Over the past decade, we have managed portfolios, acquired a primary servicer, and started an internal collection agency, resulting in a robust data set of consumer loan performance. In 2021, we began an initiative to acquire and organize borrower data across all Goal entities to enhance our internal analysis, client reporting, and to develop proprietary AI/ML predictive scoring models.
Although post-default recovery models are readily available, few options exist for early-stage delinquency models. We aimed to build a Borrower Engagement Score to predict various forms of borrower engagement, such as right party contacts or payments, to aid in default prevention efforts. Our objective is to provide servicers with information about delinquent borrowers with a higher probability of engagement so that they can improve efficiency and effectiveness by using different contact strategies for different segments. This is not a new concept for delinquent loan collections, but our unique value-add is our internal infrastructure. We developed a model using our historical data and implemented it at our internal servicer or default prevention partners on portfolios that we own or manage on behalf of our clients, without relying on third-party scoring model providers.
Why AI? One of the primary benefits of using an AI model to predict borrower behavior is the ability to analyze vast amounts of data quickly and accurately. By processing large data sets using machine learning algorithms, AI models can identify patterns and trends that may not be immediately apparent to human analysts. For example, an AI model can quickly identify correlations between borrower credit scores, employment histories, and other demographic factors, which can be used to predict the likelihood of delinquency or default. In our use case, we are predicting the likelihood that the borrower will answer the phone when dialed. The speed of AI models enables us to update the model in real-time as borrowers’ situations change daily, providing our servicing partners with updated scores daily, enabling them to change contact strategies based on near real-time data.
Once the model was built, we had to decide how to use it. How will we test it with our servicing partners? How should we change our borrower outreach as a result of the Engagement Score? How will we measure success? These decisions were a collaboration between the technology and business teams. It was decided that an initial experiment with adjustments to the outbound call strategy be conducted over a 12-week period. We would begin by only changing the outbound call strategy. After that test was completed, then we would test other omni-channel outreach strategies. We started with outbound calls to focus on one variable. To measure success, we would use cure rates and number of days to cure (to adjust for timing), promise to pay rates, and the change in Engagement Score during the period. Borrowers were segmented into two groups based on number of days delinquent: up to 60 days past due and after 60 days past due. Additionally, borrowers were segmented into groups based on Engagement Scores, along with a control group of business-as-usual outreach. This initial test would inform us of which borrowers, based on Engagement Score, to increase calls, decrease calls, or keep the status quo. This will allow our servicing partners to optimize their outreach strategies and improve efficiencies, improve cure rates, and decrease default rates.
By analyzing borrower data in real-time, the engagement model provides valuable insights into borrower preferences and behaviors including how and when they want to be contacted. Not all borrowers are the same and the model helps apply the right strategy to the right borrower to enhance customer service and engagement, which is becoming more and more important in the current economic environment.
As VP of Asset Management at Goal Solutions, Mark oversees the management of $25 billion of consumer loan portfolios on behalf of Goal’s clients which include banks, insurance companies, hedge funds, schools, and other financial stakeholders.
Mark has extensive experience managing and improving the performance of consumer loan portfolios by working with third party servicers and collection agencies. Prior to joining Goal Solutions, he was responsible for the management of over $3 billion of private student loan portfolios at Boston Portfolio Advisors (“BPA”) where he worked on client engagements involving portfolio oversight and improvement, advisory, valuation, acquisition, and sales. Prior to BPA, Mark worked at the Massachusetts Educational Financing Authority (“MEFA”) where he was part of a finance team that structured and issued over $1 billion of student loan asset-backed securities.
Education:
Northeastern University, B.S.B.A. Finance / Babson M.B.A.
https://www.linkedin.com/in/markmousseau/