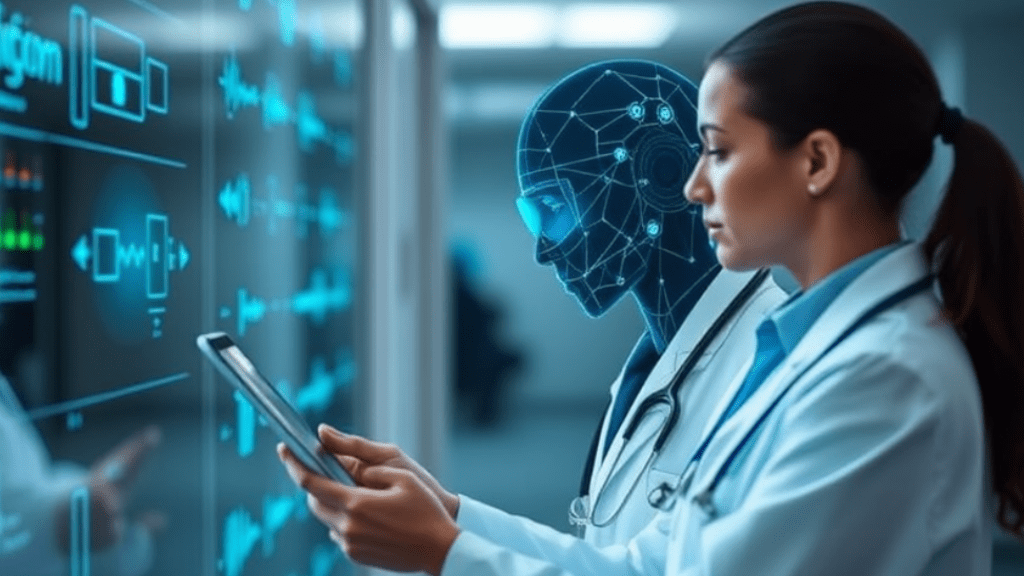
The Digital Doctor is In: AI and Telemedicine Redefine Healthcare
The healthcare industry is undergoing a seismic shift fueled by artificial intelligence (AI) and telemedicine.
Training neural networks to perform tasks such as image recognition or self-driving car navigation may one day require less computing power and hardware, thanks to a new artificial neural device developed by researchers in California at the University of San Diego. The device can perform calculations on neural networks using 100 to 1000 times less energy and space than existing CMOS-based hardware.
Generally, neural networks are a series of connected artificial neuron layers, where the output of one layer provides input for the next. The generation of this input is done by applying a mathematical calculation called a nonlinear activation function. This is a crucial part of running a neural network. But the application of this feature requires a lot of computing power and circuits because it involves the transfer of data back and forth between two separate units – memory and external processor. Researchers at UC San Diego have developed a nanometer-sized device that can effectively perform the activation function.
Neural network calculations in hardware are becoming increasingly inefficient as neural network models become larger and more complex. These researchers have developed a nanoscale device with artificial neurons that applies these computations to hardware in a very energy-efficient manner.
The new study focuses on developing hardware implementations of energy-efficient artificial neural networks. The device can implement one of the most commonly used activating functions in neural network training, called rectified linear unit. What is unique about this feature is that it needs hardware that can undergo a gradual change in resistance in order to work. And this is precisely what the researchers have designed for their device – it can gradually switch from insulating to conductive state with a bit of heat.
The switch is what’s known as a Mott transition. The architecture of this device is quite innovative and interesting. Typically, in a Mott transition, the material experiences an abrupt switch from insulating to conducting because the current flows directly through it. In this case, current flows through the nanowire at the top of the material to heat it and cause a very gradual change in resistance.
Researchers say the technology can be further expanded to perform more complex tasks such as recognizing faces and objects in self-driving cars. With the interest and cooperation of the industry, this can happen. At the moment, this is proof of the concept. This is a small system in which only one layer of the synapse is arranged with one activation layer. By collecting more of them, you can create a more complex system for different applications.
The healthcare industry is undergoing a seismic shift fueled by artificial intelligence (AI) and telemedicine.
The healthcare and pharmaceutical sectors are navigating a transformative period, with technological advancements reshaping patient care, operational efficiencies, and strategic growth.
In the world of business, financial wizards wave their wands to conjure profits and success. But behind every great money magician …
Insurtech is not just making waves in the insurance industry—it’s rewriting the rulebook. As technology-driven startups disrupt …