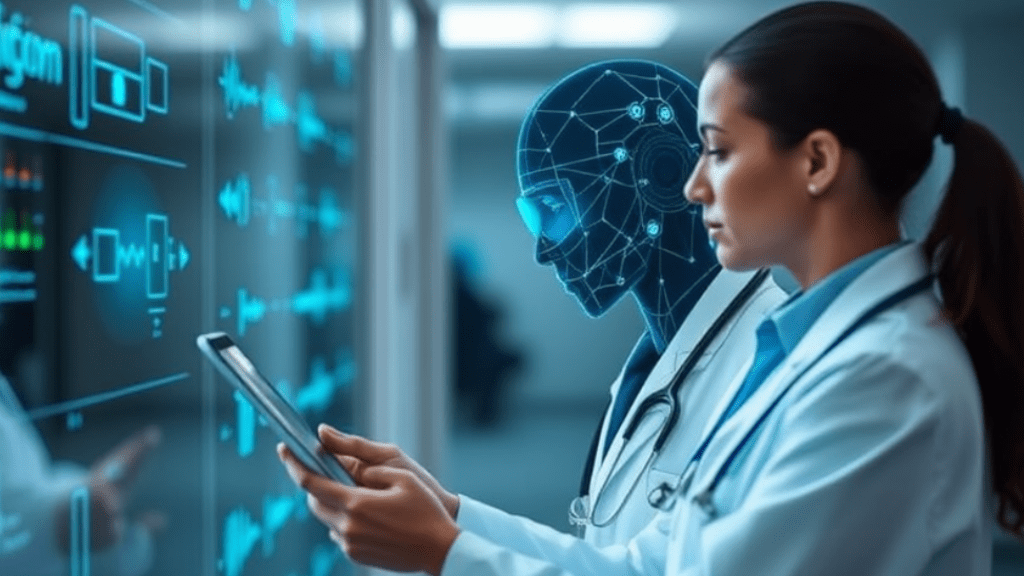
The Digital Doctor is In: AI and Telemedicine Redefine Healthcare
The healthcare industry is undergoing a seismic shift fueled by artificial intelligence (AI) and telemedicine.
A new machine-learning algorithm developed at Imperial College London can convert 2D images of composite materials into 3D structures. The algorithm can help scientists and material manufacturers to study and improve the design and production of composite materials such as battery electrodes and aircraft parts in 3D.
Utilizing data from 2D cross-sections of composite materials, which are made by combining different materials with different physical and chemical properties, the algorithm can expand the cross-sectional dimensions to convert them into 3D computerized models. This allows scientists to study other materials or “phases” of composites and how they fit.
The tool learns what 2D cross-sections of composites look like and scales them so that their phases can be studied in 3D space. In the future, it can be used to optimize the design of these types of materials, which allows scientists and manufacturers to study the layered architecture of composites.
Researchers have found that their technique is cheaper and faster than creating 3D computer representations from physical 3D objects. They have also managed to identify the different phases in the materials more clearly, which makes them more challenging to implement with modern techniques.
The combination of materials such as composites allows you to take advantage of each component’s best properties, but studying them in detail can be a challenge, as the layout of the material dramatically affects performance. The algorithm allows researchers to take their 2D images and generate 3D structures with the same properties, which will enable them to perform more realistic simulations.
Research, design, and production of composite materials in three dimensions is currently a challenge. 2D images are inexpensive to obtain and give researchers high resolution, wide field of view, and very good at telling different materials. On the other hand, 3D imaging techniques are often quite expensive and relatively blurry. Their low resolution also makes it challenging to identify the different phases in the composite.
The efficiency of many devices that contain composite materials, such as batteries, is closely related to the 3D arrangement of their components on a micro-scale. However, 3D images of these materials with sufficient detail can be complex. The researchers hope that their new machine learning tool will enable the material design community by freeing itself from dependence on expensive 3D imaging machines in many scenarios. Generation of three-dimensional structures from a two-dimensional slice with a generatively contradictory expansion of network-based dimension.
The healthcare industry is undergoing a seismic shift fueled by artificial intelligence (AI) and telemedicine.
The healthcare and pharmaceutical sectors are navigating a transformative period, with technological advancements reshaping patient care, operational efficiencies, and strategic growth.
In the world of business, financial wizards wave their wands to conjure profits and success. But behind every great money magician …
Insurtech is not just making waves in the insurance industry—it’s rewriting the rulebook. As technology-driven startups disrupt …